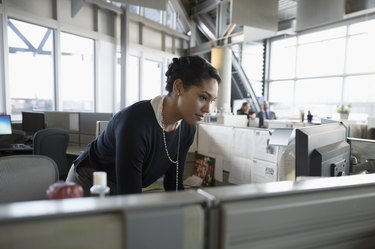
Finding a P-value in Excel for correlations is a relatively straightforward process, but unfortunately, there isn't a single Excel function for the task. Correlations are often an essential step for establishing the relationship or link between two sets of data, and you can calculate a correlation coefficient in Excel (such as Pearson's correlation coefficient) using built-in functions. There are also functions for statistical tests. However, the r-value you get for your correlation needs to be transformed into a t statistic before you can interpret the results.
Finding a Correlation Coefficient in Excel
Video of the Day
If you're looking for a Pearson correlation in Excel 2007 or a generic correlation coefficient, there are built-in functions that allow this to be calculated. First, you need two arrays of data you want to compare for correlations. Assume they're in columns A and B, running from cells 2 to 21 in each. Use the Correl or Pearson function to find the correlation coefficient in Excel. In a blank cell, either type "=Correl([array 1], [array 2])" or "=Pearson([array 1], [array 2])" to find the correlation coefficient, with the first column of data referenced where it says "[array 1]" and the second where it says "[array 2]." In the example, you'd type "=Pearson(A2:A21, B2:B21)" or "=Correl(A2:A21, B2:B21)" noting that you can also open the parenthesis and then highlight the relevant cells with your mouse or keyboard, type the comma, and then highlight the second set. This returns a correlation coefficient with a value between −1 and 1.
Video of the Day
Interpretation of a Correlation in Excel
The interpretation of a correlation in Excel crucially depends on converting the output of the correlation function into a t value. This can be done with a formula. Find a blank cell and type: "=([correlation coefficient]*SQRT([number of pairs of data]-2)/SQRT(1-[correlation coefficient]^2))" into it. Again, the square brackets represent the information you need to enter for your own specific data. For "[correlation coefficient]," enter the cell reference you used for calculating the correlation in the last section. For "[number of pairs of data]" enter the total number of data points in a single array. In the example running from cells 2 to 21 in columns A and B, there are 20 pairs of data points in total. This is n in statistical jargon. So imagine you're using the example and you put your correlation in cell C2. You'd type "=(C2 *SQRT(20-2)/SQRT(1-C2^2))" into a blank cell to find the t statistic.
Now you can use this along with the "Tdist" function to find the P-value. In another empty cell, type "=TDIST([t statistic], [degrees of freedom], [number of tails])" to perform the relevant significance test in Excel. Again, the square brackets are where you enter your specific data. The t statistic is the value you just calculated, so for the example, imagine you did this in cell C3. The degrees of freedom for a correlation is given by the sample size (n) minus two, so in the example (with n = 20), this would be 18. Finally, a one- or two-tailed test tells you whether you're looking for results in one direction or two – a positive or a negative correlation specifically. If you're not sure which direction the correlation would be in, use a two-tailed test and enter "2" in place of "[number of tails]."
In the example, you'd enter "=TDIST(C3, 18, 2)" to find the P-value. Generally, a result is considered significant if P < 0.05.
Other Versions of Excel
With newer versions of Excel, the process for finding the correlation coefficient and performing a significance test in Excel is exactly the same. The same functions exist in all later versions of Excel. However, in versions of Excel before 2003, the "Pearson" function often has rounding errors, so in older versions, you should use the "Correl" function instead.